Using Technology to Grade Assessments
- ajbachert
- Dec 6, 2021
- 6 min read
Updated: Jan 22, 2023
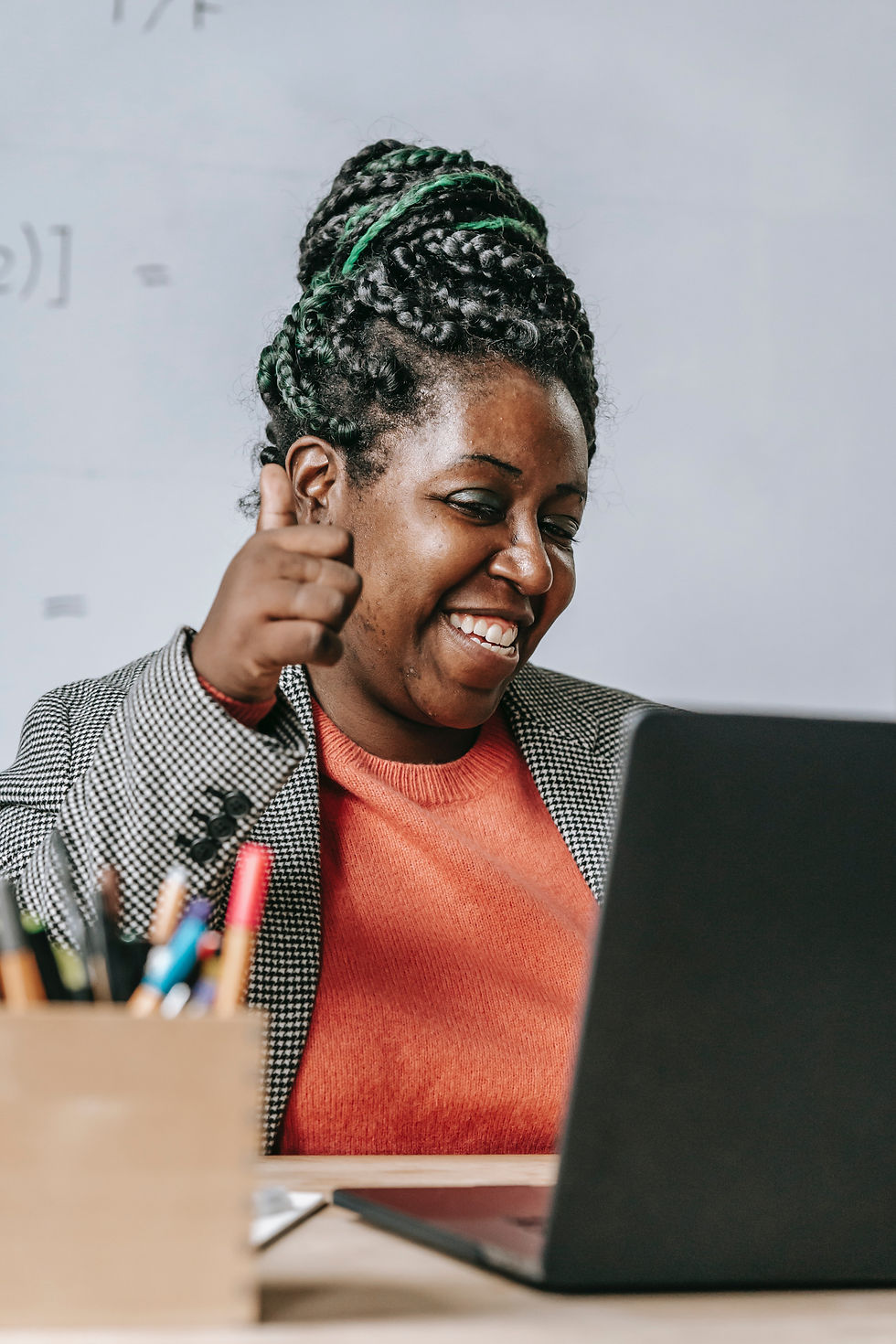
Technology has invaded almost every aspect of life. Education is no different. Technology in education can be used for communication, planning and administrative tasks, instruction and enrichment, research, and assessment. In addition to being used to assess students, technology advances are being used to score assessments of all types. Using technology to administer and grade assessments, we have more data on students than ever before, and we can use these data points to track their progress and to identify strengths and weaknesses (Cope & Kalantziz, 2016).
Beginning in 2015, Ohio began using artificial intelligence (AI) programs to score their standardized tests. As of 2018, about 75% of Ohio students’ tests were scored completely by the computer, including the written portions. The student essays are compared by the computer to model essays provided by humans. There are benefits and concerns when it comes to using AI to grade tests. Using AI provides for more fair and consistent grading, it saves time, and will eventually be a cost-saver (O’Donnell, 2018). Automated natural language processing technologies have better reliability ratings than human grading because they do not require large amounts of time and a grader with a thorough knowledge and consistent application of the rubric (Aken, 2017; Cope & Kalantzis, 2016). However, there are concerns regarding whether AI can adequately assess creativity, arguments in answers, humor, irony, imagery, idioms, metaphors, and other aspects of language (O’Donnell, 2018; Aken, 2017). There are also concerns about unclear expectations regarding the amount of material allowed to be quoted in the response, and about trusting machines to grade such high-stakes tests that can have a big impact on students (O’Donnell, 2018).
There are many types of automated assessment applications, which may make it difficult to select the right one for your purposes. Some can be used for summative scoring. Others can be used for formative assessments, and some can do both. When used to score written components, applications fall into two main groups: automated assessment and machine-assisted analysis (Aken, 2017).
Automated Assessment
Automated assessment applications only require human input for the initial set up, and they will either use text in isolation (TII) analysis (or analytical text parsing) or statistical corpus based analysis (CBA) (Aken, 2017; Cope & Kalantzis, 2016). TII can be used to analyze one or more of these areas: 1) writing style, 2) syntax, or 3) content. Writing style and syntax analysis primarily assesses the technical aspects of writing without evaluating content or factual accuracy. These things are evaluated in content analysis. These analysis methods use a variety of techniques to evaluate the writing, and they do not rely on having a large library of texts for comparison. CBA, on the other hand, compares the written sample with a collection of previously evaluated samples. CBA can be done using unordered vector analysis (UVA) or Baynesian analysis. Word choice, content, and semantics are analyzed using the CBA methods. CBA is limited in its ability to give specific feedback, and students may be able to figure out how to game the system (Aken, 2017; Cope & Kalantzis, 2016). It is not common for TII and CBA to be used together (Cope & Kalantzis, 2016).
Machine-Assisted Analysis
Machine-assisted analysis requires human interaction to provide a text analysis. This type of analysis does not provide summative scores, but it assists teachers in grading and providing feedback. There are subgroups of machine-assisted analysis such as computer-assisted feedback, and peer review (scored and un-rated). Computer-assisted systems can assist with analyzing semantics and syntax, creating and streamlining feedback, and annotations. Peer reviews allow others to collaborate, to provide feedback and annotate the original work. In scored peer reviews, a final assessment rating is included (Aken, 2017). Machine assessments can be used in other academic areas as well. Computer adaptive tests (CAT) and computer diagnostic tests (CDT) allow for immediate feedback for students, fast, accurate scores with lots of data provided to help guide and personalize instruction (Cope & Kalantzis, 2016).
Data, Data, and More Data
State standardized tests, classroom tests, college entrance exams, and many other forms of assessment are being taken and scored using technology. The more this happens, the more data is created, which could be a double-edged sword. The data could potentially be misused to profile learners and push them into certain academic tracks, to promote more teaching to the test, or for unwanted and negative surveillance. On the positive side, it could be used to personalize instruction and learning, provide responsive assessments, encourage collaborative learning, to promote more engaging pedagogy, and to do longitudinal analyses to track student growth over time (Cope & Kalantzis, 2016). Privacy concerns in regard to data are a valid issue because identities may not be protected, and guaranteeing anonymity is not possible (Cope & Kalantzis, 2016).
There are two perspectives for dealing with educational data: 1) educational data mining, and 2) learning analytics. Educational data mining gathers unstructured data, such as log files, discussion threads, key strokes in order to analyze evidences of learning. This unstructured data (not defined in predetermined model) can be used to alert teachers when a student may be disengaging and need help using devices that can capture eye movements, expressions, speech, movement, posture, etc. Learning analytics deals with more structured data gained from applications like games, simulations, and peer-review. Structured data can be used to track learning paths, provide immediate feedback, and make judgments based on reasoning using rubrics (Cope & Kalantzis, 2016).
The use of the educational data leads to more personalized learning, immediate feedback, integrated instruction and assessment, building comprehensive records and artifacts of learning, and documenting the process of learning itself. Data collection is not limited to just the teachers; students are also collecting and analyzing data. The data that is available to educators now allows them to more easily see similarities and differences between individuals, subgroups, and contributing factors to student learning (Cope & Kalantzis, 2016).
Academic Honesty
No discussion of using technology for assessments would be complete without considering online cheating. As we all know, cheating is not a new phenomenon. However, with students having access to multiple forms of technology and the internet at their fingertips, the temptation to cheat is greatly enhanced because it is easy to do. One form of cheating that I see in my classroom is when students cut and paste something directly from the internet without citing the source. They have been taught how to cite sources in English for both direct citations and paraphrased ones, and I encourage them to do so when they are using internet sources in my classroom. However, they often don’t follow through. This is a lesson that must constantly be taught and reinforced. The biggest area where I see cheating attempted is during tests. In order to prevent (or try to prevent) this, I do several things. The tests given online are not all the same, the question order and answer options are scrambled, and use Blocksi (my school’s monitoring program) to actively monitor them during the testing sessions. If I see a student trying to go to another browser to look up an answer, I close the browser and send a chat message telling them to stay on the test. Once they realize I watch what they are doing, they stop trying to do this. Students have to put up their notebooks and phones have to be put in the pocket holder on the wall prior to beginning the test. On most tests, I have at least a few subjective questions, so students have to enter their own answers. If they are cheating on those questions, it is very obvious.
In her article, How to Prevent Cheating During Online Tests, Feeney (2017), suggested many of the items I listed above. She also recommended creating a culture of honesty within the classroom, talk about academic integrity and define cheating (including all its forms), reminding them of consequences of cheating, and explaining how it affects how instruction is planned. Feeney also recommended giving shorter and more frequent quizzes to reduce the incentive to cheat (2017).
Here is a video that gives some perspective on the problem of cheating.
References
Aken, A., (2017). An evaluation of assessment-oriented computer-based text analysis paradigms. Higher Education Research, 2(4), pp. 111-116. doi: 10.11648/j.her.20170204.12
Cope, B., & Kalantzis, M. (2016). Big Data Comes to School: Implications for Learning, Assessment, and Research. AERA Open. https://doi.org/10.1177/2332858416641907
Feeney, J., (2017). How to prevent cheating during online tests. Retrieved November 8, 2018 from https://www.schoology.com/blog/how-prevent-cheating-during-online-tests
O’Donnell, P. (2018). Computers are now grading essays on Ohio's state tests. Retrieved November 9, 2018 from https://www.cleveland.com/metro/2018/03/computers_are_now_grading_essays_on_ohios_state_tests_your_ch.html
Hi Anjanette
I want to thank you for helping me see if I understood my reading correctly. It was challenging for me to understand it until it finally clicked. So, thank you. I was looking forward to reading your and Pablo's Blog. However, I like how you broke down each section and provided a good explanation of what the reading was about. That was very helpful to me. Yes, in agreement with you, a vast amount of Automated Assessment is challenging to select. I didn't realize that are so many out there, and these were only a few, and I bet there are still more to discover. All the processes and the elements required to make a program work are…